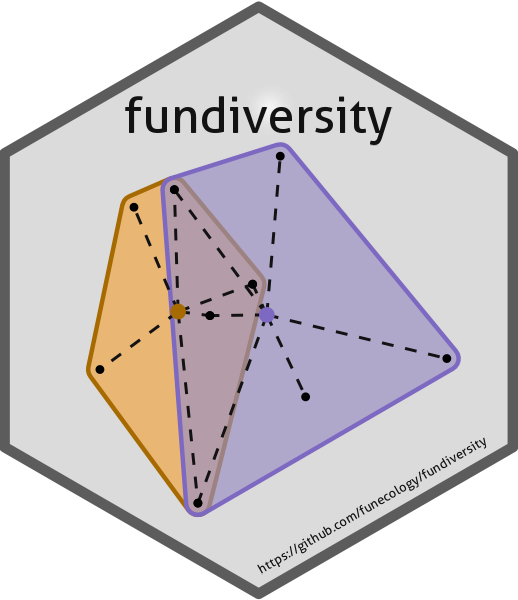
3. Performance Benchmark with other packages
Source:vignettes/fundiversity_2-performance.Rmd
fundiversity_2-performance.Rmd
This vignette presents some performance tests ran between
fundiversity
and other functional diversity packages. Note
that to avoid the dependency on other packages, this vignette is pre-computed.
Other packages to compute FD indices
Main functions
Here is a table that summarizes the comparable functions (and their
arguments) for functions included in fundiversity
. Note
that the package name is indicated before ::
followed by
the function name.
Index Type | Index Name | Source |
fundiversity function |
Equivalent Functions |
---|---|---|---|---|
α-diversity | Functional Dispersion (FDis) | Laliberté and Legendre (2010) | fd_fdis() |
BAT::dispersion() FD::fdisp() hillR::hill_func() FD for FDis computations and standardize
the traits without telling the
user)mFD::alpha.fd.multidim(..., ind_vect = "fdis")
|
α-diversity | Functional Divergence (FDiv) | Villéger, Mason, and Mouillot (2008) | fd_fdiv() |
mFD::alpha.fd.multidim(..., ind_vect = "fdiv") |
α-diversity | Functional Evenness (FEve) | Villéger, Mason, and Mouillot (2008) | fd_feve() |
mFD::alpha.fd.multidim(..., ind_vect = "feve") |
α-diversity | Functional Richness (FRic) | Villéger, Mason, and Mouillot (2008) | fd_fric() |
BAT::alpha() (tree, not strictly
equal)BAT::hull.alpha()
(hull)mFD::alpha.fd.multidim(..., ind_vect = "fric")
|
α-diversity | Rao’s Quadratic Entropy (Q) | Villéger, Grenouillet, and Brosse (2013) | fd_raoq() |
adiv::QE() BAT::rao() hillR::hill_func()
(standardize traits without
warning)mFD::alpha.fd.hill(..., q = 2, tau = "max")
(returns a slightly modified version of Q according to Ricotta and Szeidl (2009)) |
β-diversity | Functional Richness Intersection (FRic_intersect) | Rao (1982) | fd_fric_intersect() |
betapart::functional.beta.pair() hillR::hill_func_parti_pairwise()
|
The other packages are thus: adiv
(Pavoine 2020), BAT
(Cardoso, Rigal, and Carvalho 2015),
betapart
(Baselga and Orme
2012), FD
(Laliberté,
Legendre, and Shipley 2014), hillR
(Li 2018), and mFD
(Magneville et al. 2022). For fairness of
comparison, even if FD::dbFD()
contains most indices we’re
not considering it as it computes all indices together for each call,
and would necessarily be slower.
Benchmark between packages
We will now benchmark the functions included in
fundiversity
with the corresponding function in other
packages using the microbenchmark::microbenchmark()
function. We will use the fairly small (~220 species, 8 sites, 4 traits)
provided dataset in fundiversity
.
tictoc::tic() # Time execution of vignette
library(fundiversity)
data("traits_birds", package = "fundiversity")
data("site_sp_birds", package = "fundiversity")
dist_traits_birds <- dist(traits_birds)
Functional Dispersion (FDis)
fdis_bench <- microbenchmark::microbenchmark(
fundiversity = {
fundiversity::fd_fdis(traits_birds, site_sp_birds)
},
FD = {
FD::fdisp(dist_traits_birds, site_sp_birds)
},
mFD = {
mFD::alpha.fd.multidim(
traits_birds, site_sp_birds, ind_vect = "fdis",
scaling = FALSE, verbose = FALSE, details_returned = FALSE
)
},
times = 30
)
ggplot2::autoplot(fdis_bench) +
labs(title = "Functional Dispersion (FDis)")
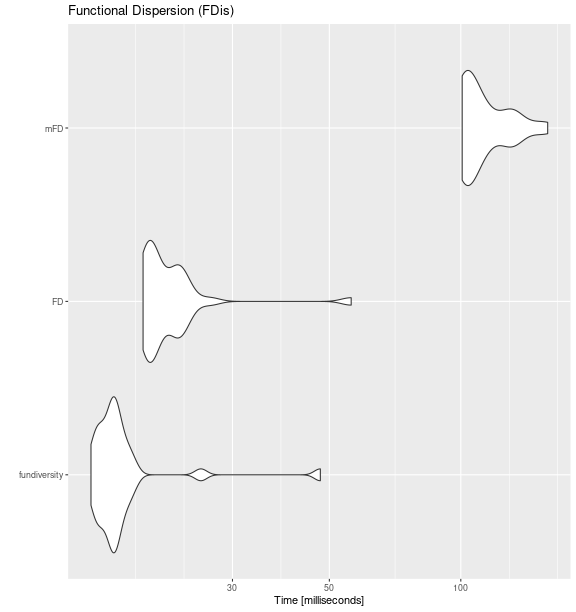
Functional Divergence (FDiv)
fdiv_bench <- microbenchmark::microbenchmark(
fundiversity = fd_fdiv(traits_birds, site_sp_birds),
mFD = mFD::alpha.fd.multidim(
traits_birds, site_sp_birds, ind_vect = "fdiv",
scaling = FALSE, verbose = FALSE
),
times = 30
)
ggplot2::autoplot(fdiv_bench) +
labs(title = "Functional Divergence (FDiv)")
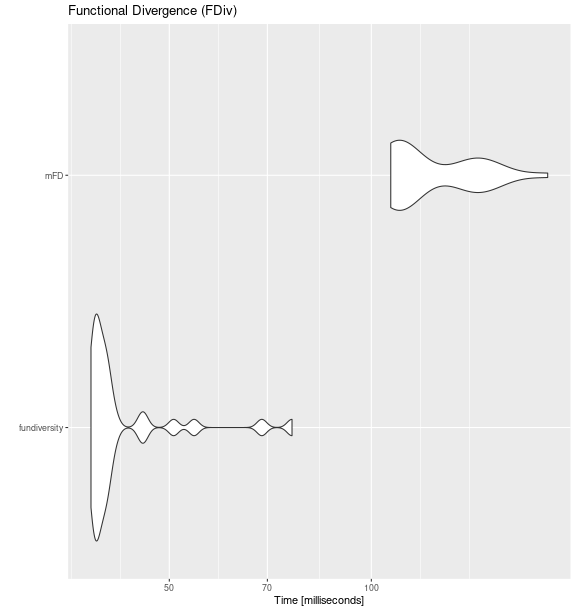
Functional Evenness (FEve)
feve_bench <- microbenchmark::microbenchmark(
fundiversity = fd_feve(traits_birds, site_sp_birds),
mFD = mFD::alpha.fd.multidim(
traits_birds, site_sp_birds, ind_vect = "feve",
scaling = FALSE, verbose = FALSE
),
times = 30
)
ggplot2::autoplot(feve_bench) +
labs(title = "Functional Evenness (FEve)")
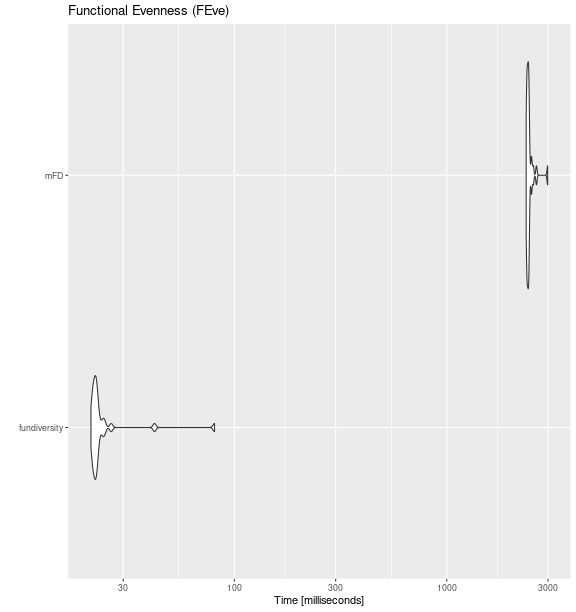
Functional Richness (FRic)
fric_bench <- microbenchmark::microbenchmark(
fundiversity = fd_fric(traits_birds, site_sp_birds),
BAT_tree = BAT::alpha(
site_sp_birds, traits_birds
),
BAT_alpha_hull = BAT::hull.alpha(
BAT::hull.build(site_sp_birds, traits_birds)
),
mFD = mFD::alpha.fd.multidim(
traits_birds, site_sp_birds, ind_vect = "fric",
scaling = FALSE, verbose = FALSE
),
times = 30
)
ggplot2::autoplot(fric_bench) +
labs(title = "Functional Richness (FRic)")
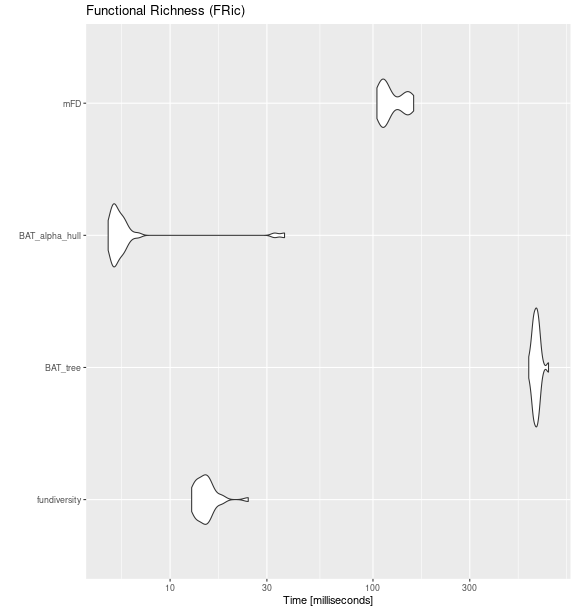
Functional Richness Intersection (FRic_intersect)
fric_bench <- microbenchmark::microbenchmark(
fundiversity = fd_fric_intersect(traits_birds, site_sp_birds) ,
betapart = betapart::functional.beta.pair(
site_sp_birds, traits_birds
),
hillR = hillR::hill_func_parti_pairwise(
site_sp_birds, traits_birds, .progress = FALSE
),
times = 30
)
ggplot2::autoplot(fric_bench) +
labs(title = "Functional Richness Intersection (FRic)")
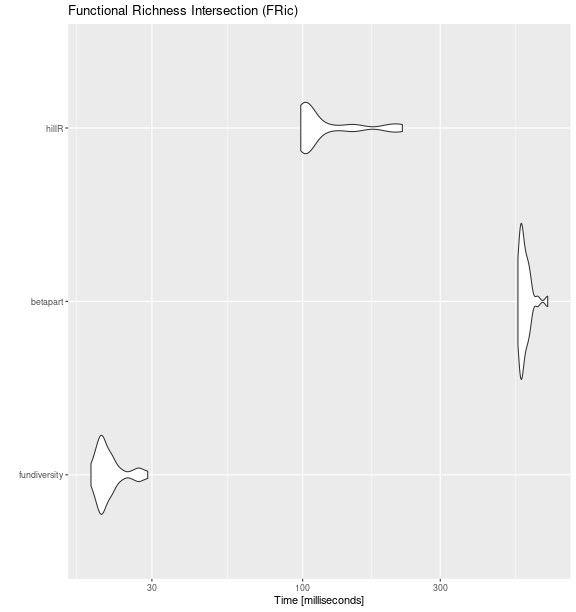
Rao’s Quadratic Entropy (Q)
raoq_bench <- fric_bench <- microbenchmark::microbenchmark(
fundiversity = fd_raoq(traits_birds, site_sp_birds),
adiv= adiv::QE(
site_sp_birds, dist_traits_birds
),
BAT_rao = BAT::rao(
site_sp_birds, distance = traits_birds
),
hillR_hill_func = hillR::hill_func(
site_sp_birds, traits_birds, fdis = FALSE
),
mFD_alpha_fd_hill = mFD::alpha.fd.hill(
site_sp_birds, dist_traits_birds, q = 2,
tau = "max"
),
times = 30
)
ggplot2::autoplot(raoq_bench) +
labs(title = "Rao's Quadatric Entropy (Q)")
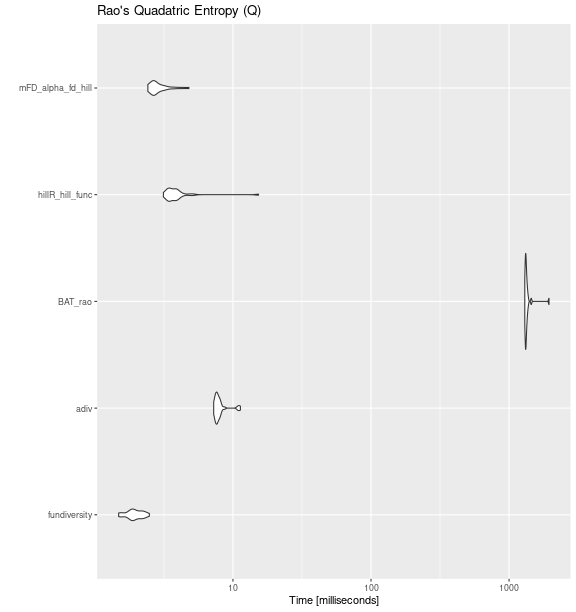
Benchmark within fundiversity
We now proceed to the performance evaluation of functions within
fundiversity
with datasets of increasing sizes.
Increasing the number of species
make_more_sp <- function(n) {
traits <- do.call(rbind, replicate(n, traits_birds, simplify = FALSE))
row.names(traits) <- paste0("sp", seq_len(nrow(traits)))
site_sp <- do.call(cbind, replicate(n, site_sp_birds, simplify = FALSE))
colnames(site_sp) <- paste0("sp", seq_len(ncol(site_sp)))
list(tr = traits, si = site_sp)
}
null_sp_1000 <- make_more_sp(5)
null_sp_10000 <- make_more_sp(50)
null_sp_100000 <- make_more_sp(500)
Functional Richness
bench_sp_fric <- microbenchmark::microbenchmark(
species_200 = fd_fric( traits_birds, site_sp_birds),
species_1000 = fd_fric( null_sp_1000$tr, null_sp_1000$si),
species_10000 = fd_fric( null_sp_10000$tr, null_sp_10000$si),
species_100000 = fd_fric(null_sp_100000$tr, null_sp_100000$si),
times = 30
)
ggplot2::autoplot(bench_sp_fric)
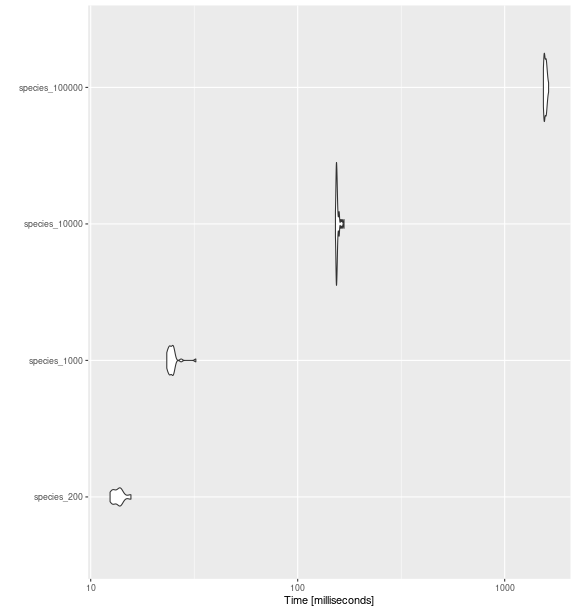
fd_fric()
with increasing number of species.
bench_sp_fric
#> Unit: milliseconds
#> expr min lq mean median uq max neval cld
#> species_200 12.40461 12.86473 13.63794 13.65822 13.96949 15.64313 30 a
#> species_1000 23.31024 23.98235 24.77462 24.52898 24.99526 32.20389 30 b
#> species_10000 152.16972 153.69475 155.65197 154.41463 155.87235 167.39586 30 c
#> species_100000 1538.24210 1552.02784 1573.05390 1571.92017 1587.70159 1629.76043 30 d
Functional Divergence
bench_sp_fdiv <- microbenchmark::microbenchmark(
species_200 = fd_fdiv( traits_birds, site_sp_birds),
species_1000 = fd_fdiv( null_sp_1000$tr, null_sp_1000$si),
species_10000 = fd_fdiv( null_sp_10000$tr, null_sp_10000$si),
species_100000 = fd_fdiv(null_sp_100000$tr, null_sp_100000$si),
times = 30
)
ggplot2::autoplot(bench_sp_fdiv)
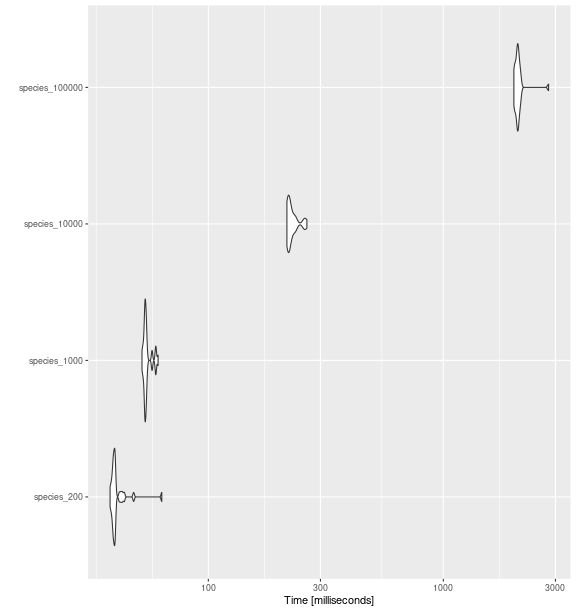
fd_fdiv()
with increasing number of species.
bench_sp_fdiv
#> Unit: milliseconds
#> expr min lq mean median uq max neval cld
#> species_200 38.18281 39.33241 41.03782 39.80735 40.19474 63.38092 30 a
#> species_1000 52.21140 53.58856 54.88775 54.00482 54.58979 61.12244 30 a
#> species_10000 215.96505 218.21261 227.32449 221.46767 231.33971 262.64920 30 b
#> species_100000 1996.22764 2035.16809 2090.07993 2068.75306 2097.02749 2805.37816 30 c
Rao’s Quadratic Entropy
bench_sp_raoq <- microbenchmark::microbenchmark(
species_200 = fd_raoq( traits_birds, site_sp_birds),
species_1000 = fd_raoq( null_sp_1000$tr, null_sp_1000$si),
species_10000 = fd_raoq( null_sp_10000$tr, null_sp_10000$si),
times = 30
)
ggplot2::autoplot(bench_sp_raoq)
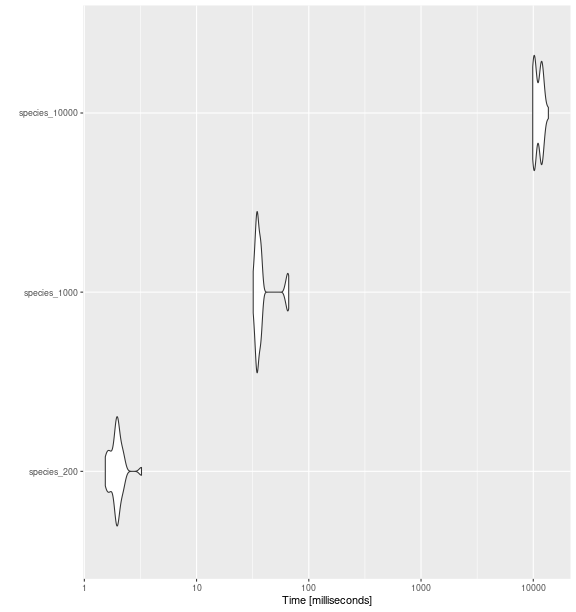
fd_raoq()
with increasing number of species.
bench_sp_raoq
#> Unit: milliseconds
#> expr min lq mean median uq max neval cld
#> species_200 1.537703 1.751935 1.954434 1.950017 2.041037 3.230497 30 a
#> species_1000 31.957110 34.274255 39.128452 35.156704 37.556228 66.194976 30 a
#> species_10000 9890.756358 10163.144236 11121.366410 11055.979331 11964.694993 13632.668874 30 b
Functional Evenness
bench_sp_feve <- microbenchmark::microbenchmark(
species_200 = fd_feve( traits_birds, site_sp_birds),
species_1000 = fd_feve( null_sp_1000$tr, null_sp_1000$si),
species_10000 = fd_feve( null_sp_10000$tr, null_sp_10000$si),
times = 15
)
ggplot2::autoplot(bench_sp_feve)
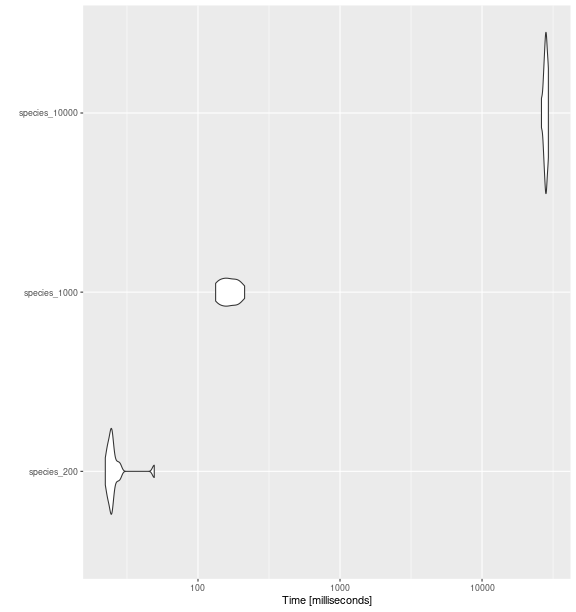
fd_feve()
with increasing number of species.
bench_sp_feve
#> Unit: milliseconds
#> expr min lq mean median uq max neval cld
#> species_200 22.25434 23.58857 26.28398 24.66652 25.41282 49.21613 15 a
#> species_1000 133.12192 148.39296 167.29508 163.98844 186.30949 212.94652 15 a
#> species_10000 26209.90991 27553.75296 28085.39492 28186.57578 28599.03918 29311.05335 15 b
Comparing between indices
all_bench_sp <- list(fric = bench_sp_fric,
fdiv = bench_sp_fdiv,
raoq = bench_sp_raoq,
feve = bench_sp_feve) %>%
bind_rows(.id = "fd_index") %>%
mutate(n_sp = gsub("species_", "", expr) %>%
as.numeric())
all_bench_sp %>%
ggplot(aes(n_sp, time * 1e-9, color = fd_index)) +
geom_point(alpha = 1/3) +
geom_smooth() +
scale_x_log10() +
scale_y_log10() +
scale_color_brewer(type = "qual",
labels = c(fric = "FRic", fdiv = "FDiv", raoq = "Rao's Q",
feve = "FEve")) +
labs(title = "Performance comparison between indices",
x = "# of species", y = "Time (in seconds)",
color = "FD index") +
theme_bw() +
theme(aspect.ratio = 1)
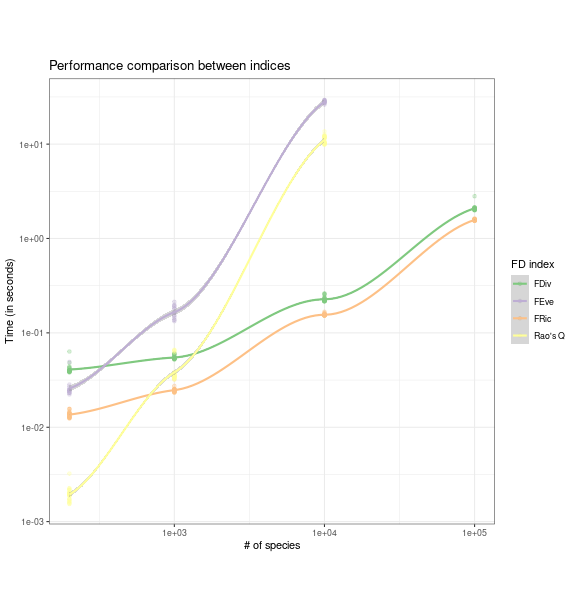
fundiversity
with increasing number of
species. Smoothed trend lines and standard error envelopes ares
shown.Increasing the number of sites
make_more_sites <- function(n) {
site_sp <- do.call(rbind, replicate(n, site_sp_birds, simplify = FALSE))
rownames(site_sp) <- paste0("s", seq_len(nrow(site_sp)))
site_sp
}
site_sp_100 <- make_more_sites(12)
site_sp_1000 <- make_more_sites(120)
site_sp_10000 <- make_more_sites(1200)
Functional Richness
bench_sites_fric <- microbenchmark::microbenchmark(
sites_10 = fd_fric(traits_birds, site_sp_birds),
sites_100 = fd_fric(traits_birds, site_sp_100),
sites_1000 = fd_fric(traits_birds, site_sp_1000),
sites_10000 = fd_fric(traits_birds, site_sp_10000),
times = 15
)
ggplot2::autoplot(bench_sites_fric)
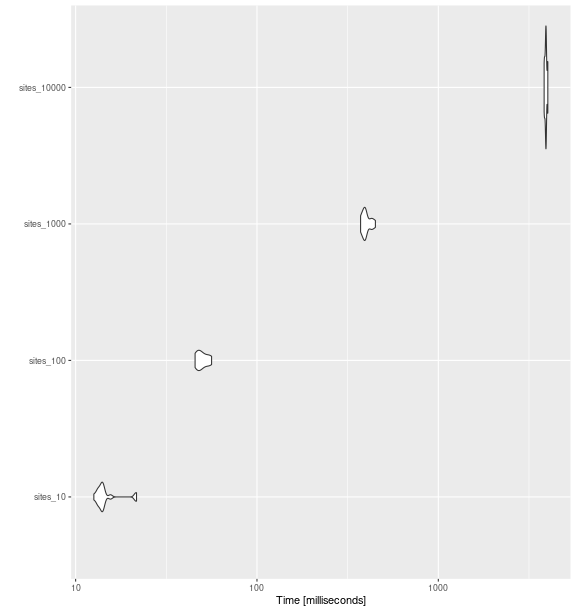
fd_fric()
with increasing number of sites.
bench_sites_fric
#> Unit: milliseconds
#> expr min lq mean median uq max neval cld
#> sites_10 12.60195 13.45944 14.88054 13.96007 14.29602 21.66510 15 a
#> sites_100 45.57530 47.25923 49.87524 48.75620 52.29567 56.19952 15 b
#> sites_1000 372.72833 386.35628 400.43169 395.60903 408.99573 449.87164 15 c
#> sites_10000 3833.05136 3885.11306 3921.60544 3919.29055 3942.32492 4016.96576 15 d
Functional Divergence
bench_sites_fdiv <- microbenchmark::microbenchmark(
sites_10 = fd_fdiv(traits_birds, site_sp_birds),
sites_100 = fd_fdiv(traits_birds, site_sp_100),
sites_1000 = fd_fdiv(traits_birds, site_sp_1000),
sites_10000 = fd_fdiv(traits_birds, site_sp_10000),
times = 15
)
ggplot2::autoplot(bench_sites_fdiv)
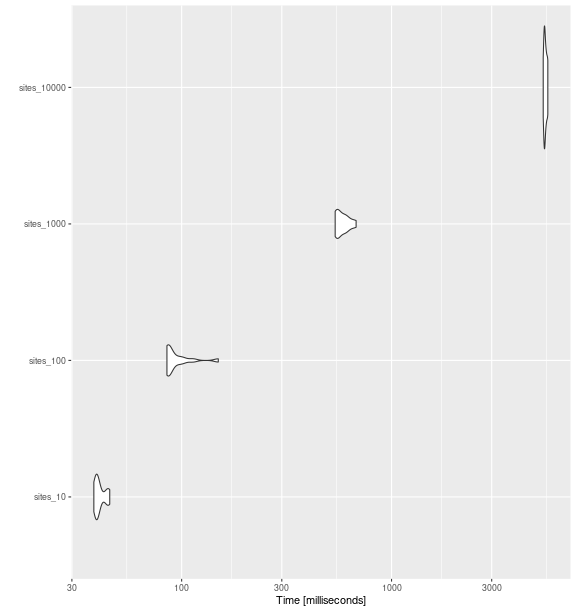
fd_fdiv()
with increasing number of sites.
bench_sites_fdiv
#> Unit: milliseconds
#> expr min lq mean median uq max neval cld
#> sites_10 38.17040 39.17880 40.79484 39.59029 42.08936 45.43952 15 a
#> sites_100 85.09805 85.99399 94.77048 86.88365 96.33675 149.45931 15 b
#> sites_1000 538.76135 545.03628 582.84610 564.85683 605.87486 677.86382 15 c
#> sites_10000 5278.55172 5336.69296 5405.90861 5378.53730 5458.24597 5552.26594 15 d
Rao’s Quadratic Entropy
bench_sites_raoq = microbenchmark::microbenchmark(
sites_10 = fd_raoq(traits = NULL, site_sp_birds, dist_traits_birds),
sites_100 = fd_raoq(traits = NULL, site_sp_100, dist_traits_birds),
sites_1000 = fd_raoq(traits = NULL, site_sp_1000, dist_traits_birds),
sites_10000 = fd_raoq(traits = NULL, site_sp_10000, dist_traits_birds),
times = 15
)
ggplot2::autoplot(bench_sites_raoq)
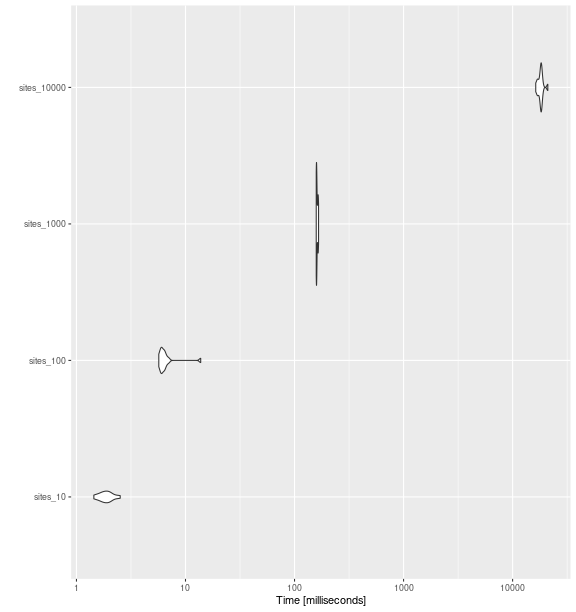
fd_raoq()
with increasing number of sites.
bench_sites_raoq
#> Unit: milliseconds
#> expr min lq mean median uq max neval cld
#> sites_10 1.443757 1.717630 1.887153 1.861230 2.037991 2.518933 15 a
#> sites_100 5.689849 6.004428 6.718675 6.178415 6.460348 13.786219 15 a
#> sites_1000 158.130064 158.814848 160.907719 159.296631 163.977418 166.066294 15 a
#> sites_10000 16296.391504 17530.369693 18080.130808 18193.538354 18411.571843 21033.249202 15 b
Functional Evenness
bench_sites_feve <- microbenchmark::microbenchmark(
sites_10 = fd_feve(traits = NULL, site_sp_birds, dist_traits_birds),
sites_100 = fd_feve(traits = NULL, site_sp_100, dist_traits_birds),
sites_1000 = fd_feve(traits = NULL, site_sp_1000, dist_traits_birds),
sites_10000 = fd_feve(traits = NULL, site_sp_10000, dist_traits_birds),
times = 15
)
ggplot2::autoplot(bench_sites_feve)
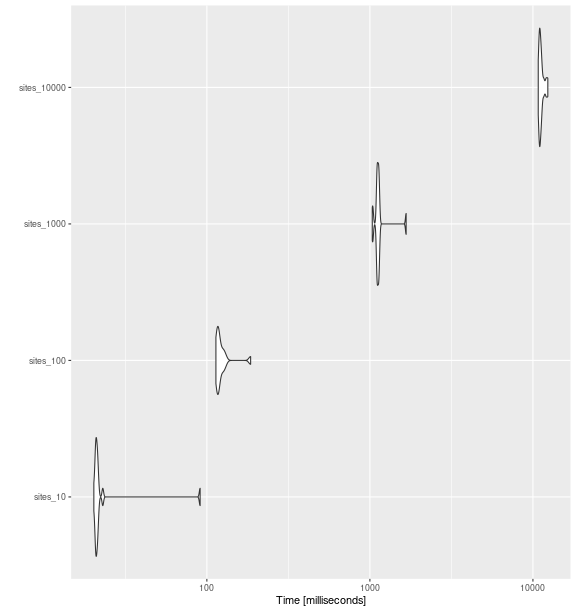
fd_feve()
with increasing number of sites
bench_sites_feve
#> Unit: milliseconds
#> expr min lq mean median uq max neval cld
#> sites_10 20.26885 20.79379 25.80265 21.06491 21.39182 90.92815 15 a
#> sites_100 113.64419 115.81009 123.60943 118.04145 123.54457 185.57582 15 a
#> sites_1000 1034.37573 1107.36408 1146.78358 1119.68000 1134.07348 1668.68480 15 b
#> sites_10000 10779.65515 10969.94333 11249.27062 11090.00824 11317.37080 12341.22800 15 c
Comparing between indices
all_bench_sites <- list(fric = bench_sites_fric,
fdiv = bench_sites_fdiv,
raoq = bench_sites_raoq,
feve = bench_sites_feve) %>%
bind_rows(.id = "fd_index") %>%
mutate(n_sites = gsub("sites", "", expr) %>%
as.numeric())
all_bench_sites %>%
ggplot(aes(n_sites, time * 1e-9, color = fd_index)) +
geom_point(alpha = 1/3) +
geom_smooth() +
scale_x_log10() +
scale_y_log10() +
scale_color_brewer(type = "qual",
labels = c(fric = "FRic", fdiv = "FDiv", raoq = "Rao's Q",
feve = "FEve")) +
labs(title = "Performance comparison between indices",
x = "# of sites", y = "Time (in seconds)",
color = "FD index") +
theme_bw() +
theme(aspect.ratio = 1)
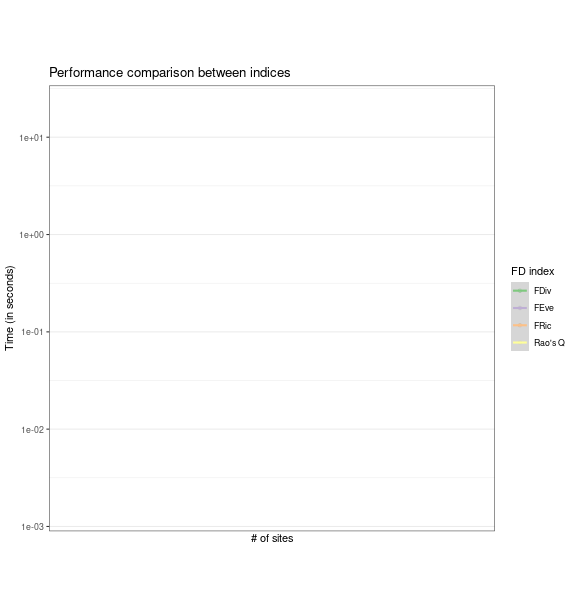
fundiversity
with increasing number of
sites. Smoothed trend lines and standard error envelopes ares
shown.Session info of the machine on which the benchmark was ran and time it took to run
#> seconds needed to generate this document: 1691.449 sec elapsed
#> ─ Session info ─────────────────────────────────────────────────────────────────────────────────────────────────────────────────────────────────
#> setting value
#> version R version 4.2.1 (2022-06-23)
#> os Ubuntu 20.04.5 LTS
#> system x86_64, linux-gnu
#> ui RStudio
#> language (EN)
#> collate en_US.UTF-8
#> ctype en_US.UTF-8
#> tz Etc/UTC
#> date 2022-11-15
#> rstudio 2022.02.0+443 Prairie Trillium (server)
#> pandoc 2.17.1.1 @ /usr/lib/rstudio-server/bin/quarto/bin/ (via rmarkdown)
#>
#> ─ Packages ─────────────────────────────────────────────────────────────────────────────────────────────────────────────────────────────────────
#> package * version date (UTC) lib source
#> abind 1.4-5 2016-07-21 [1] CRAN (R 4.2.0)
#> ade4 1.7-19 2022-04-19 [1] CRAN (R 4.2.0)
#> adegraphics 1.0-16 2021-09-16 [1] CRAN (R 4.2.1)
#> adiv 2.2 2022-10-06 [1] CRAN (R 4.2.1)
#> ape 5.6-2 2022-03-02 [1] CRAN (R 4.2.0)
#> assertthat 0.2.1 2019-03-21 [3] CRAN (R 4.1.3)
#> base64enc 0.1-3 2015-07-28 [1] CRAN (R 4.2.1)
#> BAT 2.9.2 2022-11-08 [1] CRAN (R 4.2.1)
#> betapart 1.5.6 2022-04-06 [1] CRAN (R 4.2.1)
#> cachem 1.0.6 2021-08-19 [3] CRAN (R 4.1.3)
#> caret 6.0-93 2022-08-09 [1] CRAN (R 4.2.1)
#> class 7.3-20 2022-01-13 [5] CRAN (R 4.1.2)
#> cli 3.4.1 2022-09-23 [1] CRAN (R 4.2.1)
#> cluster 2.1.3 2022-03-28 [5] CRAN (R 4.1.3)
#> clusterGeneration 1.3.7 2020-12-15 [1] CRAN (R 4.2.0)
#> coda 0.19-4 2020-09-30 [1] CRAN (R 4.2.0)
#> codetools 0.2-18 2020-11-04 [5] CRAN (R 4.0.3)
#> colorspace 2.0-3 2022-02-21 [1] CRAN (R 4.2.0)
#> combinat 0.0-8 2012-10-29 [1] CRAN (R 4.2.0)
#> crayon 1.5.1 2022-03-26 [1] CRAN (R 4.2.0)
#> data.table 1.14.2 2021-09-27 [1] CRAN (R 4.2.0)
#> DBI 1.1.2 2021-12-20 [3] CRAN (R 4.1.3)
#> deldir 1.0-6 2021-10-23 [1] CRAN (R 4.2.1)
#> dendextend 1.16.0 2022-07-04 [1] CRAN (R 4.2.1)
#> digest 0.6.29 2021-12-01 [1] CRAN (R 4.2.0)
#> doParallel 1.0.17 2022-02-07 [1] CRAN (R 4.2.1)
#> doSNOW 1.0.20 2022-02-04 [1] CRAN (R 4.2.1)
#> dplyr * 1.0.10 2022-09-01 [1] CRAN (R 4.2.1)
#> e1071 1.7-12 2022-10-24 [1] CRAN (R 4.2.1)
#> ellipsis 0.3.2 2021-04-29 [1] CRAN (R 4.2.0)
#> evaluate 0.18 2022-11-07 [1] CRAN (R 4.2.1)
#> expm 0.999-6 2021-01-13 [1] CRAN (R 4.2.0)
#> fansi 1.0.3 2022-03-24 [1] CRAN (R 4.2.0)
#> farver 2.1.0 2021-02-28 [1] CRAN (R 4.2.0)
#> fastcluster 1.2.3 2021-05-24 [1] CRAN (R 4.2.1)
#> fastmap 1.1.0 2021-01-25 [1] CRAN (R 4.2.1)
#> fastmatch 1.1-3 2021-07-23 [1] CRAN (R 4.2.0)
#> FD 1.0-12.1 2022-05-02 [1] CRAN (R 4.2.0)
#> foreach 1.5.2 2022-02-02 [1] CRAN (R 4.2.1)
#> fundiversity * 0.2.1.9000 2022-04-12 [3] Github (bisaloo/fundiversity@87652ba)
#> future 1.28.0 2022-09-02 [1] CRAN (R 4.2.1)
#> future.apply 1.10.0 2022-11-05 [1] CRAN (R 4.2.1)
#> generics 0.1.2 2022-01-31 [1] CRAN (R 4.2.0)
#> geometry 0.4.6 2022-04-18 [1] CRAN (R 4.2.0)
#> ggplot2 * 3.3.6 2022-05-03 [1] CRAN (R 4.2.0)
#> globals 0.16.1 2022-08-28 [1] CRAN (R 4.2.1)
#> glue 1.6.2 2022-02-24 [1] CRAN (R 4.2.0)
#> gower 1.0.0 2022-02-03 [1] CRAN (R 4.2.1)
#> gridExtra 2.3 2017-09-09 [1] CRAN (R 4.2.1)
#> gtable 0.3.0 2019-03-25 [1] CRAN (R 4.2.0)
#> hardhat 1.2.0 2022-06-30 [1] CRAN (R 4.2.1)
#> here 1.0.1 2020-12-13 [3] CRAN (R 4.1.3)
#> highr 0.9 2021-04-16 [1] CRAN (R 4.2.1)
#> hillR 0.5.1 2021-03-02 [1] CRAN (R 4.2.0)
#> hms 1.1.1 2021-09-26 [1] CRAN (R 4.2.0)
#> htmltools 0.5.3 2022-07-18 [1] CRAN (R 4.2.1)
#> htmlwidgets 1.5.4 2021-09-08 [1] CRAN (R 4.2.1)
#> httr 1.4.4 2022-08-17 [1] CRAN (R 4.2.1)
#> hypervolume 3.0.4 2022-05-28 [1] CRAN (R 4.2.1)
#> igraph 1.3.2 2022-06-13 [1] CRAN (R 4.2.0)
#> interp 1.1-3 2022-07-13 [1] CRAN (R 4.2.1)
#> ipred 0.9-13 2022-06-02 [1] CRAN (R 4.2.1)
#> iterators 1.0.14 2022-02-05 [1] CRAN (R 4.2.1)
#> itertools 0.1-3 2014-03-12 [1] CRAN (R 4.2.1)
#> jpeg 0.1-9 2021-07-24 [1] CRAN (R 4.2.1)
#> jsonlite 1.8.3 2022-10-21 [1] CRAN (R 4.2.1)
#> KernSmooth 2.23-20 2021-05-03 [5] CRAN (R 4.0.5)
#> knitr 1.40 2022-08-24 [1] CRAN (R 4.2.1)
#> ks 1.13.5 2022-04-14 [1] CRAN (R 4.2.1)
#> lattice 0.20-45 2021-09-22 [3] CRAN (R 4.1.3)
#> latticeExtra 0.6-30 2022-07-04 [1] CRAN (R 4.2.1)
#> lava 1.7.0 2022-10-25 [1] CRAN (R 4.2.1)
#> lifecycle 1.0.3 2022-10-07 [1] CRAN (R 4.2.1)
#> listenv 0.8.0 2019-12-05 [1] CRAN (R 4.2.1)
#> lpSolve 5.6.15 2020-01-24 [1] CRAN (R 4.2.0)
#> lubridate 1.9.0 2022-11-06 [1] CRAN (R 4.2.1)
#> magic 1.6-0 2022-02-09 [1] CRAN (R 4.2.0)
#> magrittr 2.0.3 2022-03-30 [1] CRAN (R 4.2.0)
#> maps 3.4.0 2021-09-25 [1] CRAN (R 4.2.0)
#> MASS 7.3-58.1 2022-08-03 [3] CRAN (R 4.2.1)
#> Matrix 1.4-1 2022-03-23 [3] CRAN (R 4.1.3)
#> mclust 6.0.0 2022-10-31 [1] CRAN (R 4.2.1)
#> memoise 2.0.1 2021-11-26 [3] CRAN (R 4.1.3)
#> mFD 1.0.2 2022-11-08 [1] CRAN (R 4.2.1)
#> mgcv 1.8-40 2022-03-29 [5] CRAN (R 4.1.3)
#> microbenchmark 1.4.9 2021-11-09 [3] CRAN (R 4.1.3)
#> mnormt 2.1.0 2022-06-07 [1] CRAN (R 4.2.0)
#> ModelMetrics 1.2.2.2 2020-03-17 [1] CRAN (R 4.2.1)
#> multcomp 1.4-19 2022-04-26 [1] CRAN (R 4.2.0)
#> munsell 0.5.0 2018-06-12 [1] CRAN (R 4.2.0)
#> mvtnorm 1.1-3 2021-10-08 [1] CRAN (R 4.2.0)
#> nlme 3.1-159 2022-08-09 [3] CRAN (R 4.2.1)
#> nls2 0.3-3 2022-05-02 [1] CRAN (R 4.2.1)
#> nnet 7.3-17 2022-01-13 [5] CRAN (R 4.1.2)
#> numDeriv 2016.8-1.1 2019-06-06 [1] CRAN (R 4.2.0)
#> palmerpenguins 0.1.1 2022-08-15 [1] CRAN (R 4.2.1)
#> parallelly 1.32.1 2022-07-21 [1] CRAN (R 4.2.1)
#> patchwork 1.1.2 2022-08-19 [1] CRAN (R 4.2.1)
#> pdist 1.2.1 2022-05-02 [1] CRAN (R 4.2.1)
#> permute 0.9-7 2022-01-27 [1] CRAN (R 4.2.0)
#> phangorn 2.9.0 2022-06-16 [1] CRAN (R 4.2.0)
#> phylobase 0.8.10 2020-03-01 [1] CRAN (R 4.2.1)
#> phytools 1.0-3 2022-04-05 [1] CRAN (R 4.2.0)
#> picante 1.8.2 2020-06-10 [1] CRAN (R 4.2.1)
#> pillar 1.7.0 2022-02-01 [1] CRAN (R 4.2.0)
#> pkgconfig 2.0.3 2019-09-22 [1] CRAN (R 4.2.0)
#> plotrix 3.8-2 2021-09-08 [1] CRAN (R 4.2.0)
#> plyr 1.8.7 2022-03-24 [1] CRAN (R 4.2.0)
#> png 0.1-7 2013-12-03 [1] CRAN (R 4.2.1)
#> pracma 2.4.2 2022-09-22 [1] CRAN (R 4.2.1)
#> prettyunits 1.1.1 2020-01-24 [1] CRAN (R 4.2.0)
#> pROC 1.18.0 2021-09-03 [1] CRAN (R 4.2.1)
#> prodlim 2019.11.13 2019-11-17 [1] CRAN (R 4.2.1)
#> progress 1.2.2 2019-05-16 [1] CRAN (R 4.2.0)
#> proto 1.0.0 2016-10-29 [1] CRAN (R 4.2.1)
#> proxy 0.4-27 2022-06-09 [1] CRAN (R 4.2.1)
#> purrr 0.3.4 2020-04-17 [1] CRAN (R 4.2.0)
#> quadprog 1.5-8 2019-11-20 [1] CRAN (R 4.2.0)
#> R6 2.5.1 2021-08-19 [1] CRAN (R 4.2.0)
#> raster 3.6-3 2022-09-18 [1] CRAN (R 4.2.1)
#> rcdd 1.5 2021-11-18 [1] CRAN (R 4.2.1)
#> RColorBrewer 1.1-3 2022-04-03 [1] CRAN (R 4.2.0)
#> Rcpp 1.0.8.3 2022-03-17 [1] CRAN (R 4.2.0)
#> recipes 1.0.3 2022-11-09 [1] CRAN (R 4.2.1)
#> reshape2 1.4.4 2020-04-09 [1] CRAN (R 4.2.1)
#> rgeos 0.5-9 2021-12-15 [1] CRAN (R 4.2.1)
#> rgl 0.110.2 2022-09-26 [1] CRAN (R 4.2.1)
#> rlang 1.0.6 2022-09-24 [1] CRAN (R 4.2.1)
#> rmarkdown 2.13 2022-03-10 [3] CRAN (R 4.1.3)
#> rncl 0.8.6 2022-03-18 [1] CRAN (R 4.2.1)
#> RNeXML 2.4.8 2022-10-19 [1] CRAN (R 4.2.1)
#> rpart 4.1.16 2022-01-24 [5] CRAN (R 4.1.2)
#> rprojroot 2.0.3 2022-04-02 [1] CRAN (R 4.2.1)
#> rstudioapi 0.14 2022-08-22 [1] CRAN (R 4.2.1)
#> sandwich 3.0-2 2022-06-15 [1] CRAN (R 4.2.0)
#> scales 1.2.0 2022-04-13 [1] CRAN (R 4.2.0)
#> scatterplot3d 0.3-41 2018-03-14 [1] CRAN (R 4.2.0)
#> sessioninfo 1.2.2 2021-12-06 [3] CRAN (R 4.1.3)
#> snow 0.4-4 2021-10-27 [1] CRAN (R 4.2.1)
#> sp 1.5-0 2022-06-05 [1] CRAN (R 4.2.0)
#> stringi 1.7.6 2021-11-29 [1] CRAN (R 4.2.0)
#> stringr 1.4.0 2019-02-10 [1] CRAN (R 4.2.0)
#> survival 3.3-1 2022-03-03 [3] CRAN (R 4.1.3)
#> terra 1.6-17 2022-09-10 [1] CRAN (R 4.2.1)
#> TH.data 1.1-1 2022-04-26 [1] CRAN (R 4.2.0)
#> tibble 3.1.7 2022-05-03 [1] CRAN (R 4.2.0)
#> tictoc 1.0.1 2021-04-19 [3] CRAN (R 4.1.3)
#> tidyr 1.2.1 2022-09-08 [1] CRAN (R 4.2.1)
#> tidyselect 1.2.0 2022-10-10 [1] CRAN (R 4.2.1)
#> timechange 0.1.1 2022-11-04 [1] CRAN (R 4.2.1)
#> timeDate 4021.106 2022-09-30 [1] CRAN (R 4.2.1)
#> utf8 1.2.2 2021-07-24 [1] CRAN (R 4.2.0)
#> uuid 1.1-0 2022-04-19 [1] CRAN (R 4.2.1)
#> vctrs 0.5.0 2022-10-22 [1] CRAN (R 4.2.1)
#> vegan 2.6-2 2022-04-17 [1] CRAN (R 4.2.0)
#> viridis 0.6.2 2021-10-13 [1] CRAN (R 4.2.1)
#> viridisLite 0.4.0 2021-04-13 [1] CRAN (R 4.2.0)
#> withr 2.5.0 2022-03-03 [1] CRAN (R 4.2.0)
#> xfun 0.34 2022-10-18 [1] CRAN (R 4.2.1)
#> XML 3.99-0.12 2022-10-28 [1] CRAN (R 4.2.1)
#> xml2 1.3.3 2021-11-30 [1] CRAN (R 4.2.1)
#> yaml 2.3.6 2022-10-18 [1] CRAN (R 4.2.1)
#> zoo 1.8-10 2022-04-15 [1] CRAN (R 4.2.0)
#>
#> [1] /home/ke76dimu/R-library/4.2
#> [2] /usr/local/lib/R/site-library
#> [3] /data/library/4.1
#> [4] /usr/lib/R/site-library
#> [5] /usr/lib/R/library
#>
#> ────────────────────────────────────────────────────────────────────────────────────────────────────────────────────────────────────────────────